We have published a new paper “Efficient Processing of Streaming Data using Multiple Abstractions” at the IEEE Cloud 2021 conference. (to be available at https://conferences.computer.org/cloud/2021/)
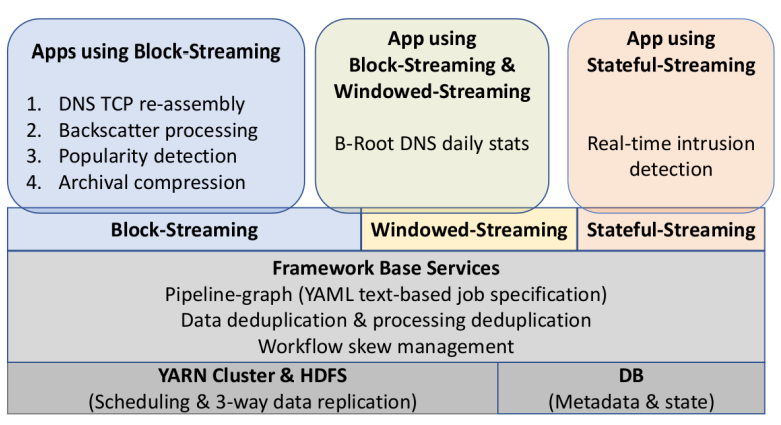
From the abstract of our paper:
Large websites and distributed systems employ sophisticated analytics to evaluate successes to celebrate and problems to be addressed. As analytics grow, different teams often require different frameworks, with dozens of packages supporting with streaming and batch processing, SQL and no-SQL. Bringing multiple frameworks to bear on a large, changing dataset often create challenges where data transitions—these impedance mismatches can create brittle glue logic and performance problems that consume developer time. We propose Plumb, a meta-framework that can bridge three different abstractions to meet the needs of a large class of applications in a common workflow. Large-block streaming (Block-Streaming) is suitable for single-pass applications that care about the temporal and spatial locality. Windowed-Streaming allows applications to process a group of data and many reductions. Stateful-Streaming enables applications to keep a long-term state and always-on behavior. We show that it is possible to bridge abstractions, with a common, high-level workflow specification, while the system transitions data batch processing and block- and record-level streaming as required. The challenge in bridging abstractions is to minimize latency while allowing applications to select between sequential and parallel operation, while handling out-of-order data delivery, component failures, and providing clear semantics in the face of missing data. We demonstrate these abstractions evaluating a 10-stage workflow of DNS analytics that has been in production use with Plumb for 2 years, comparing to a brittle hand-built system that has run for more than 3 years.
This conference paper is joint work of Abdul Qadeer and John Heidemann from USC/ISI.
Plumb is open source software and will be available at: https://ant.isi.edu/software/plumb/index.html
Update 2021-09-26: This paper was given a “special paper award” at IEEE Conference on Cloud Computing 2021! Congratulations, Abdul!